Can AI take over usability testing? We put it to the test
Curious about whether AI could offer reliable design feedback, we conducted a study with ChatGPT, prompting it to evaluate UX aspects of various prototypes. While it provided broad insights, ChatGPT fell short in identifying specific usability issues, often focusing on design over actual user interactions. Our findings reveal that while AI feedback can be a helpful starting point, it cannot replace the unique insights gained from real user testing.
Background
The rapid development of AI had a significant impact on various professional fields, including UX research. AI research tools are increasingly used to analyze user behavior, find patterns, and even generate design suggestions. These AI-driven insights complement traditional UX research methods. They can offer new ideas, and speed up the research process. However, similarly to other professions, there is also a concern among researchers about AI replacing them.
Some people also suggest that AI couldn’t just replace researchers, but also the participants of traditional research methods. This is called a synthetic user. As NN defines, “A synthetic user is an AI-generated profile that attempts to mimic a user group, providing artificial research findings produced without studying real users. A synthetic user will express simulated thoughts, needs, and experiences.”
So can AI really replace human testers in UX research? We put ChatGPT to the test to find out.

ChatGPT 4 is a multimodal large language model created by OpenAI. Since it’s multimodal, you can upload images to it, and ask for feedback about the visuals. This was the starting point for our study as well.
We started playing around with ChatGPT by uploading a few independent screens to explore the possibilities. We found that ChatGPT can give you feedback about the designs from a usability and accessibility perspective. It can also provide improvement suggestions about the content and design.
After trying this out with simple screens, the natural question came up: would this work for prototypes too? We quickly found out that ChatGPT couldn’t process Figma links, so we can’t directly send a prototype to it. However, we found a workaround: uploading a screenshot of the screens that build up a flow. Based on our experience, ChatGPT can detect the flows from these screenshots quite well, and it can rate them from a usability and accessibility perspective.
With this in mind, we wanted to see how much we can actually rely on ChatGPT for design feedback. More importantly, we wanted to compare it to input received from real users during usability testing to see how reliable ChatGPT’s suggestions were.
How we conducted our experiment
Step 1 - choosing study materials
As we needed to compare ChatGPT’s output with findings from actual usability tests, we needed to choose user flows that we had previously tested. As an agency, we have worked on multiple different projects over the years, so we had plenty of options to choose from. Since data privacy is important to us, we only used materials from clients who gave us their permission.
We wanted to use multiple scenarios for the study to be able to generalize our findings a bit more. The idea was to see how well GPT's suggestions hold up depending on how complex and specific the designs and the products are.
To do this, we decided to use three different types of prototypes:
- A website that is accessible to almost everyone, both on desktop and mobile.
- A web app, but with a niche target audience. We used a compliance software application for this purpose.
- A mobile app with a specific target audience. We ended up using an early prototype for a robotics programming software that targets children between the ages of 8 and 16.
In the case of former clients, we reached out to ask for their approval to upload the designs to ChatGPT.
Step 2 - data preparation
We had to ensure the data was organized in a uniform way that ChatGPT could work with. To achieve this, we collected all the screens of the specific flows.
In some cases, we needed to make certain adjustments to make sure ChatGPT could recognize what was happening on the given screen. An example of this would be making sure that if there are pop ups or overlays, they are also included.
We created a version with labeled screens and one without labels. We wanted to see if this influences the results and if ChatGPT can still figure out the flows without the screen labels. We also collected all the usability findings and the research questions from the tests in the same FigJam board to have everything at one place. You can see part of our FigJam board below that contains all the information about IKEA.
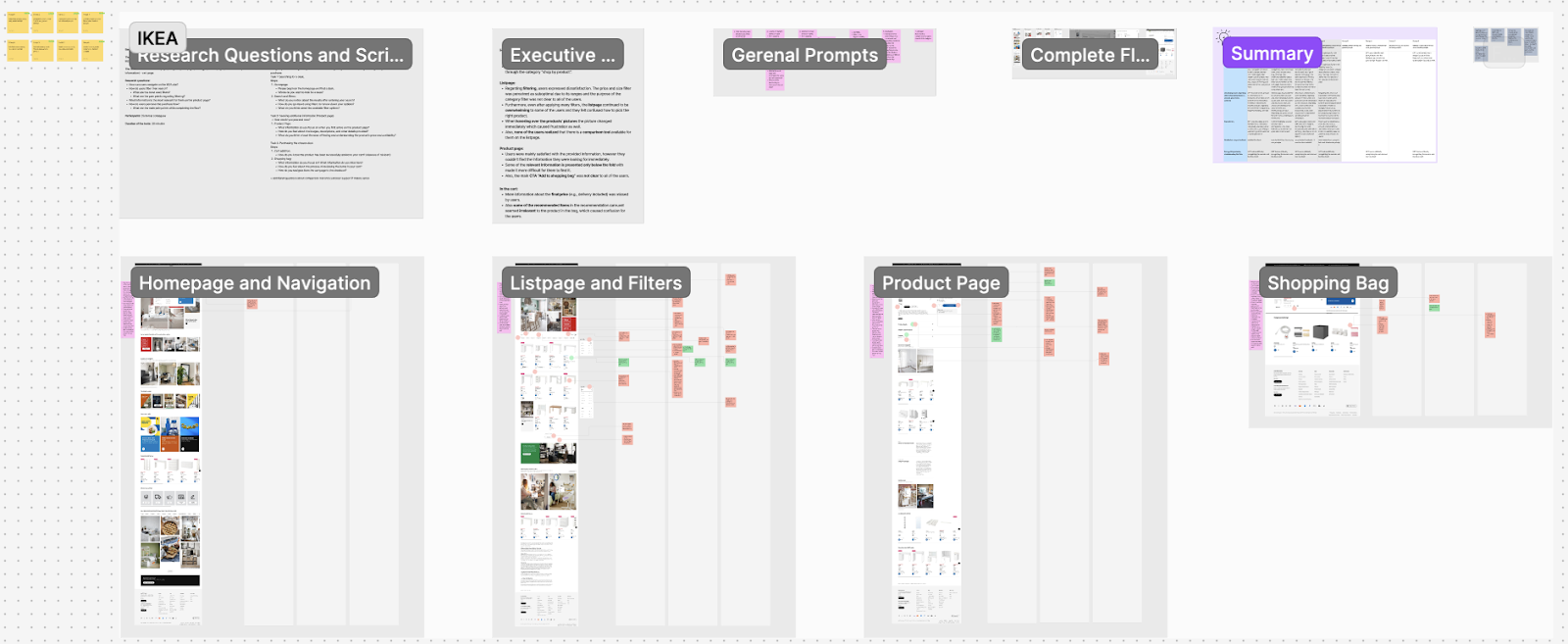
Step 3 - prompting
The next step was to create the prompts for ChatGPT. In the prompts, we tired to include the original research questions so we can compare ChatGPT's output to the actual user feedback. To make sure our findings are as useful as possible, we also tried out several different prompts for a single prototype.
Some of the prompts were more general, meaning we asked ChatGPT to collect all the usability issues. This is one of the prompts we used: "In the following test, I will show you a flow on IKEA's (UK) website. Walk me through all the usability issues that you discover on each screen and throughout the whole flow as well."
Other prompts we created were much more detailed. We included all the questions that researchers used when they were testing the prototypes with real users. Here is an example of such a detailed prompt: “What can you see on this screen? What do you think this pop-up is about? How would you proceed? What do you expect to happen after selecting the skill level?”
We also needed to give a bit of context for ChatGPT in the prompts, especially with more complicated prototypes. With the Revolution Robotics prototype, we mentioned for example that the target audience is children between the ages of 8 and 16.
Besides using different prompts, we also varied how we uploaded the screens - in some cases, we sent one screenshot showing all the screens of the flow. We also tried uploading the screens one by one to see if that made a difference.
Step 4 - analyzing the output
As the last step, we needed to compare the output with our usability test findings. We also needed to compare the output of the different prompts with each other. Below is an example of how we documented our findings in comparison tables in FigJam.

Step 5 - synthesizing insights
The fifth and final step was to find common patterns between the ChatGPT outputs we got for the different prototypes and prompts. We discussed our results together and drew conclusions based on our findings. Finally, we collected our biggest learnings and suggestions based on these.
Biggest learnings
Now that you know how we conducted the study, let’s move on to the exciting part - what we learned from it. It’s important to note we primarily used ChatGPT for this experiment, but we also replicated some of the prompts with other large language models, and the results were very similar. Based on this, we believe that our learnings could be generalized to other LLMs as well.
The feedback is general rather than specific
One thing we found is that ChatGPT rarely provides anything specific. It usually talks about design-related issues in a very general way. Overall, the feedback you get from it is very different from what you would see when monitoring real user behavior.
Example #1
As an example, see this feedback that we received from ChatGPT about one of the prototypes:
“Users might not know what certain buttons or icons do without proper labeling or instructions.”
It mentions inconsistent icons but never tells you what the specific inconsistencies are. This is a major difference compared to usability tests, where we see exactly what elements of the prototype participants struggle with.
Example #2
Another example would be this output from ChatGPT:
“Complex Navigation: The screens seem to have a lot of different paths or options for a user to choose from, which could be overwhelming for children, especially younger ones in the target age group.”
Again, this is a very general finding. During an actual user test, we would see exactly where children get stuck. Based on usability tests, we’d also have an idea about the actual mental model of the participants: how they imagine the product to work, their expectations, and goals. This is something we’re completely missing from ChatGPT’s answer.
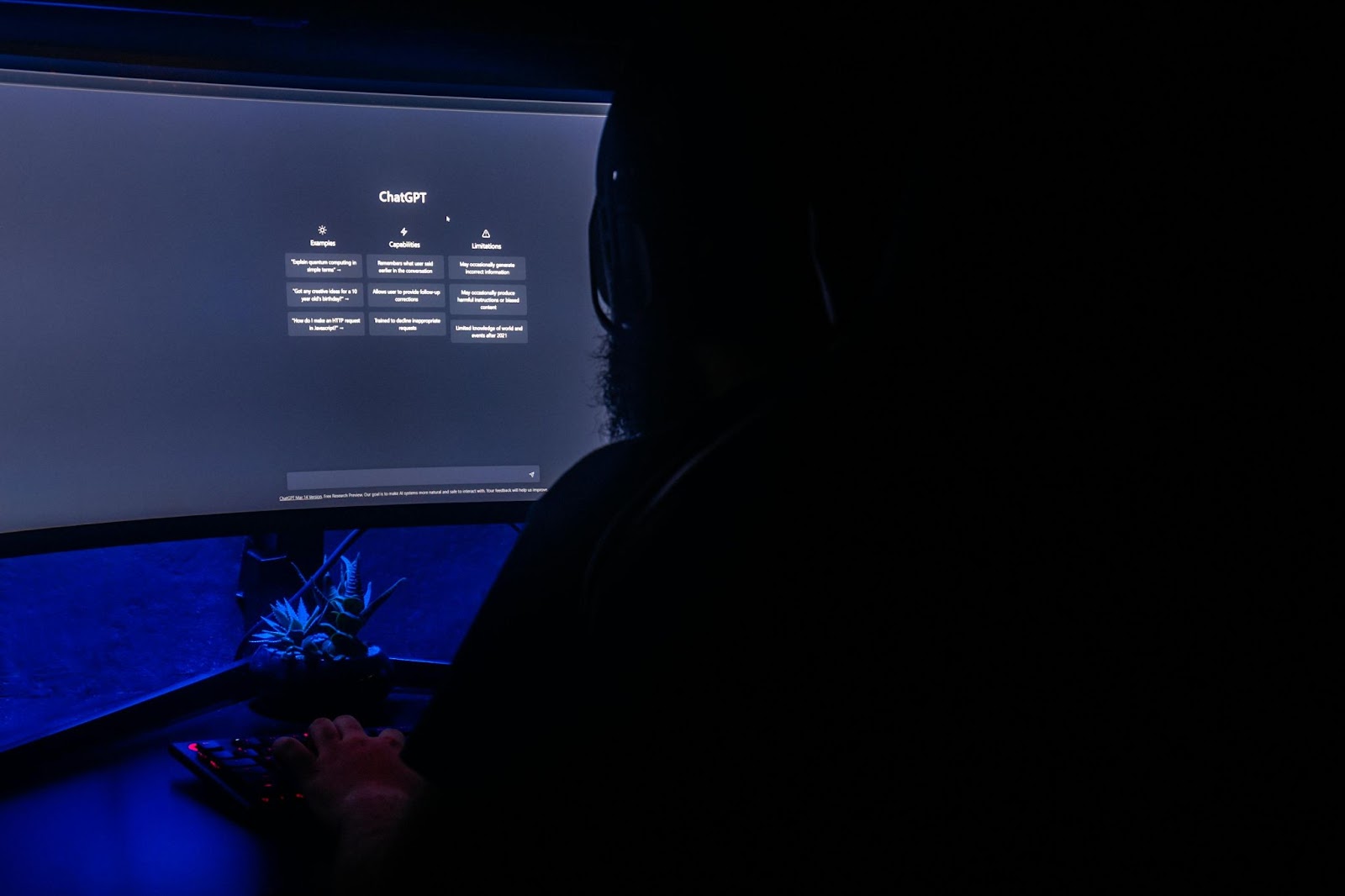
ChatGPT can’t tell you anything about interactions
As ChatGPT can only work from screenshots of screens that make up a flow, it can’t tell you which interactions work well and which don’t, as opposed to usability tests.
Example
For example, when testing one of the prototypes, we could see that participants didn’t realize that they could scroll the sidebar. They also interacted with some elements in ways that we didn’t expect them to: instead of simply tapping, they tried to tap and hold to move them around. However, ChatGPT didn’t realize that these behaviors might occur with any of the prompts.
The feedback is focused on overall design as opposed to usability
The feedback you get from ChatGPT is very design-focused and fundamentally different from talking to actual users, even when you prompt it to answer as a real user would. In many cases, it didn’t recognize big usability issues that we could identify with tests.
Example
One good example of this was when the majority of test participants couldn’t find the rename and delete options in the prototype. However, ChatGPT never realized this issue and when we asked it to think like an actual user, the problem still didn’t come up: it recognized which buttons could be used for renaming or deleting, even when real target audience members couldn’t.
There were minor differences based on labeling and prompts
Overall, there weren’t big differences between labeled and unlabeled designs. In some cases, labeling helped ChatGPT to get a clearer understanding of what the screen is about. But even without labels, it could usually recognize the purpose of the different screens.
As we mentioned before, we used several prompts to be able to compare these. The main difference was between general and detailed prompts. ChatGPT gave a much shorter answer when we asked it to collect all usability issues compared to when we used all the questions that were in the original usability scripts. However, just because the answer was longer, it didn’t necessarily mean it was better. ChatGPT was often rambling and explaining minor details behind the answers that weren’t useful to us.
The same was true for uploading all the screens in a single screenshot or one by one. When we uploaded each screen separately, the response was longer and more detailed. However, we didn’t notice an improvement in the quality of the answers.
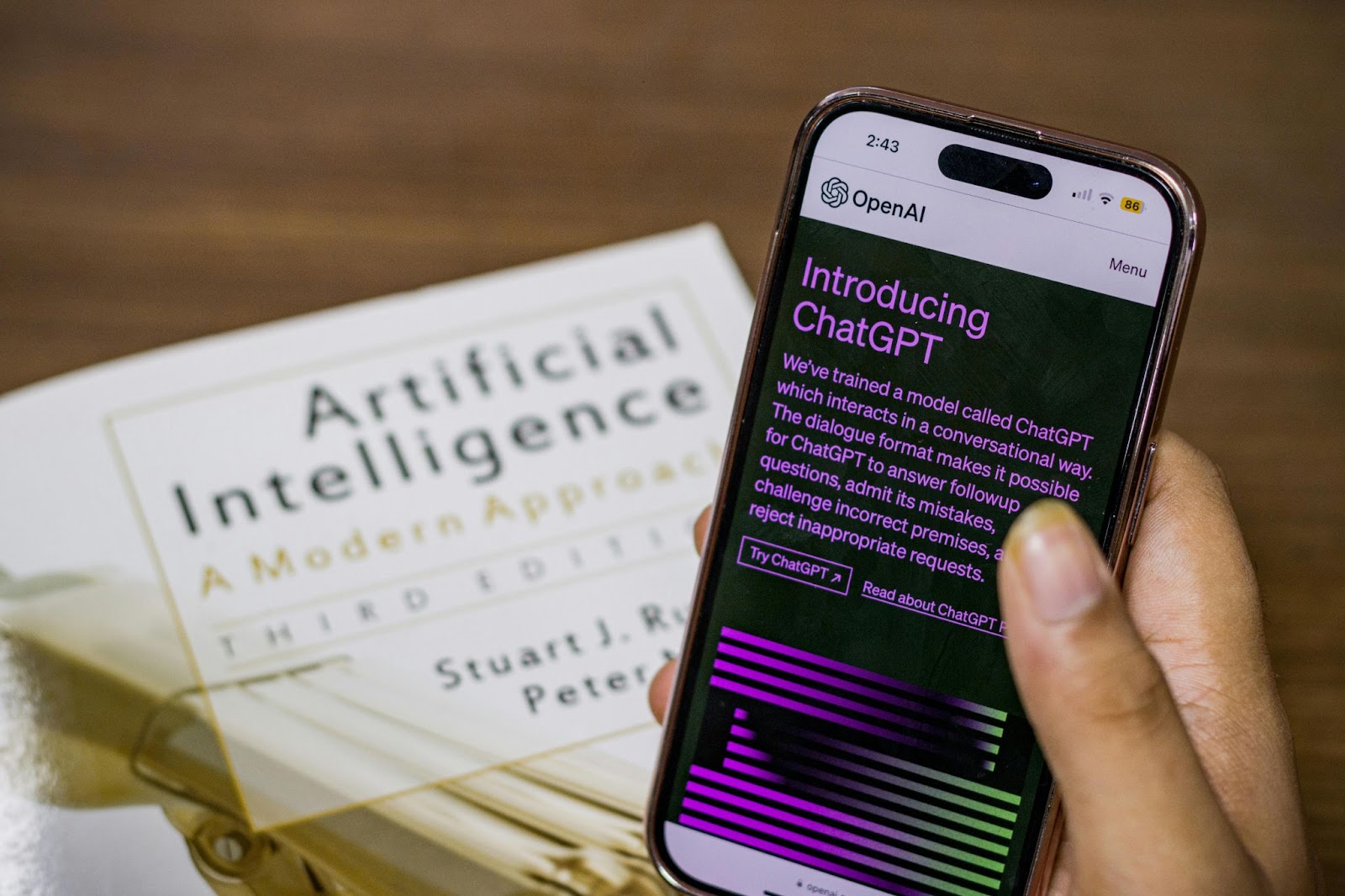
Contradictions in the feedback are quite common
It’s a well-known fact that ChatGPT is prone to fabrication. Even when it can’t clearly answer a question, it still tries. Sometimes, this results in false information. That happened in this study as well.
Example #1
There were several cases when ChatGPT gave contradictory design feedback. For example, we asked it to collect all the positive and negative aspects of the designs. Consistent design was mentioned as a positive thing, while it also talked about design inconsistencies among the negative design attributes.
Example #2
In the case of the IKEA website, we also received confusing feedback from ChatGPT about the visuals.
As positive: “The use of IKEA's recognizable branding, colors, and design elements makes the website visually appealing.”
As negative: “The page is overcrowded with various content blocks, promotions, and visuals, making it difficult for users to focus on specific tasks or find what they need quickly.”
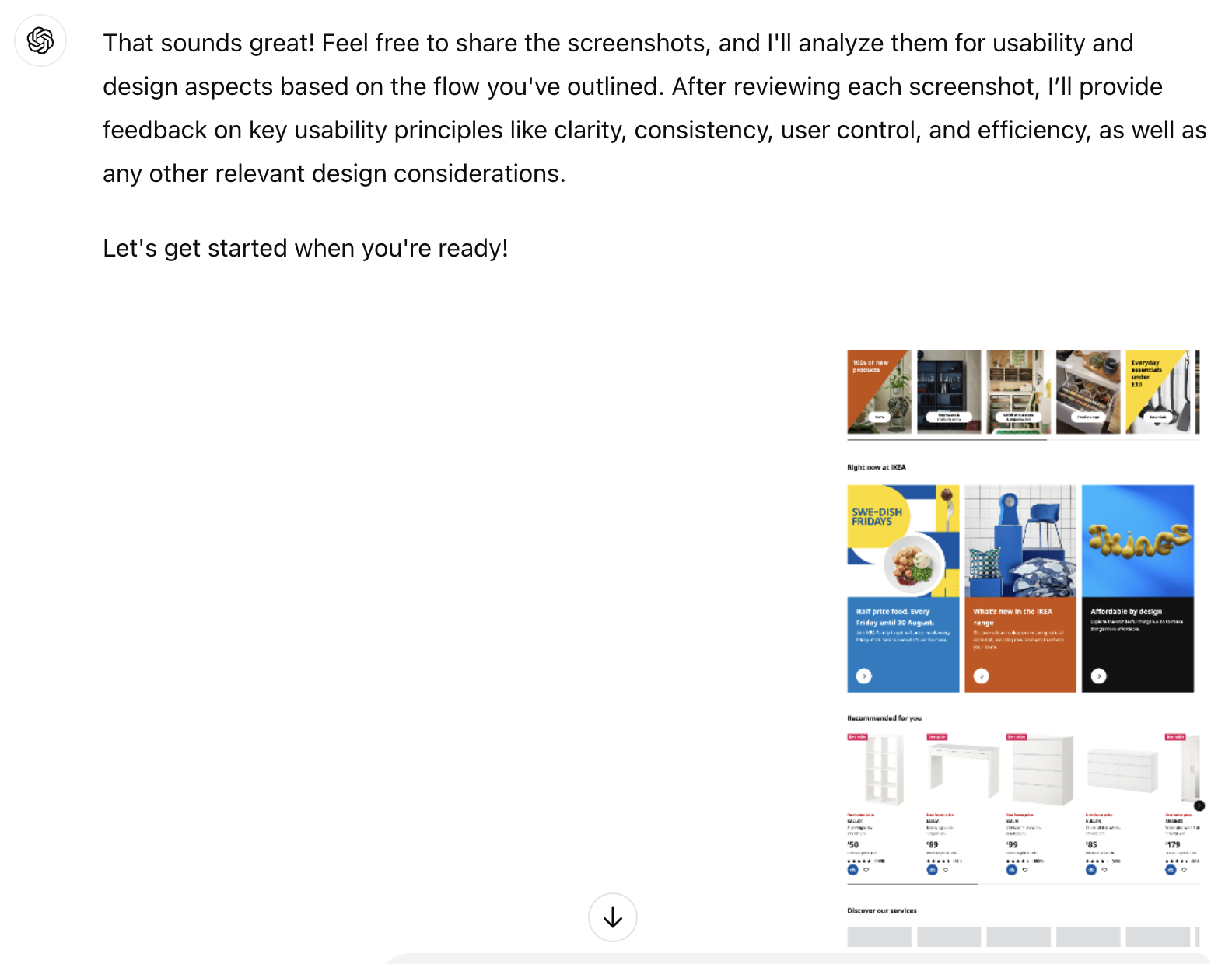
Recommendations
Now that we looked at some of the key learnings from the study, let’s see our recommendations. Based on the findings, you should keep 3 things in mind when using ChatGPT for UX design feedback:
- Use it only for general design feedback, not as a replacement for usability tests. It can give you a couple of ideas, but it can’t tell you what real members of your product’s target audience would do. Especially, if you have a special or niche target audience - like children in our case with the Revolution Robotics prototype.
- Double check the answers and don’t take its recommendations blindly. As explained earlier, it can hallucinate and contradict itself.
- Try to ask about the details for better findings. If you ask generally about design consistency, it might leave out important information. If you ask specifically about buttons, you have a better chance that it will mention inconsistencies about buttons.
Example prompt
Here is an example about how to prompt ChatGPT for the best possible design feedback. All of these questions are part of one conversation that starts from general inquiry and continuously gets narrower.
- Give feedback about the website's UX/UI design.
- Keep the following design principles in mind: UI, Aesthetics, Readability, Visual Hierarchy, Navigation, and Design Consistency.
- Summarize what is good and what is bad on the screens. List reasons why.
- Provide general design suggestions and also specific ones to each principle.
- From a UX design perspective, what do you think about the call to action buttons in this user flow?
As you can see, this prompt focuses on general UX design feedback and principles, and not usability issues. However, even in this case, you shouldn’t accept ChatGPT’s answers without critically assessing them.
Summary
While ChatGPT offers valuable insights for UX design, our study shows that it cannot replace usability testing with actual target audience members. Although it can provide useful design feedback and inspire improvements, ChatGPT struggles to accurately simulate human perspectives or consistently identify major usability issues.
Key limitations include:
- Inability to truly adopt user perspectives: When prompted to answer as a target audience member, ChatGPT attempts but fails to authentically replicate human thought processes.
- Inconsistent issue identification: ChatGPT often misses critical usability problems.
- Potential for misinformation: As ChatGPT can produce "hallucinations" or false information, all its feedback requires careful verification.
- Lack of nuanced understanding: The AI cannot capture the depth of insight gained from observing real users interacting with a product.
In conclusion, ChatGPT can be a helpful tool for generating ideas and initial design feedback. However, it can’t replace traditional usability testing with human participants. We believe that our findings could be used as a strong argument against synthetic users. The results of our study show that synthetic research can’t provide reliable data for usability testing purposes.